Published
- 5 min read
AI Facts 3
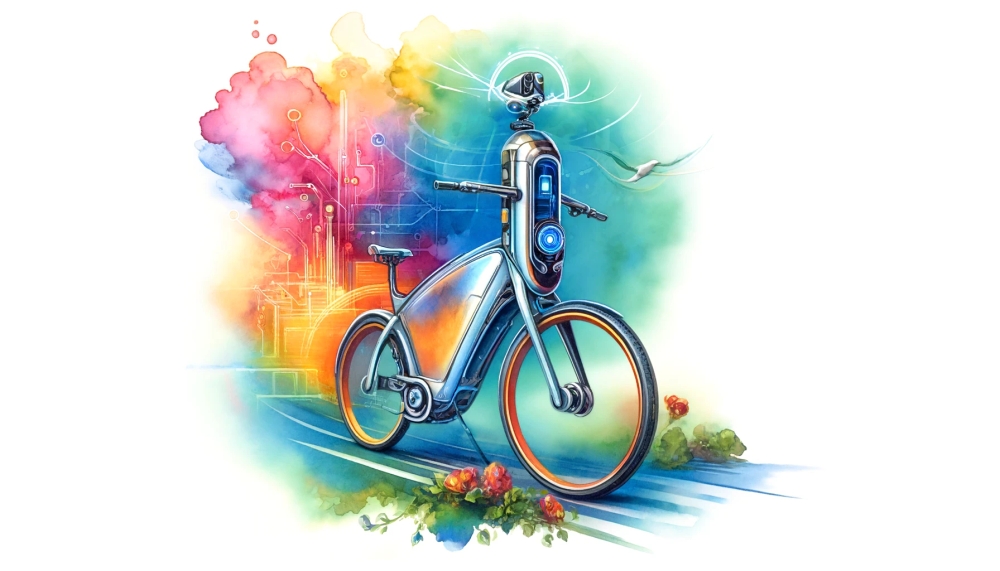
Backpropagation
- Backpropagation is a popular algorithm used in training artificial neural networks.
- The algorithm works by calculating the gradient of the loss function with respect to the weights of the network and then updating the weights using gradient descent.
- Backpropagation is an iterative process that involves propagating the error backwards through the network, adjusting the weights at each layer to minimize the error.
import numpy as np
# Define the sigmoid activation function
def sigmoid(x):
return 1 / (1 + np.exp(-x))
# Define the derivative of the sigmoid function
def sigmoid_derivative(x):
return x * (1 - x)
# Define the neural network class
class NeuralNetwork:
def __init__(self, inputs, hidden, outputs):
self.inputs = inputs
self.hidden = hidden
self.outputs = outputs
self.weights_ih = np.random.rand(self.inputs, self.hidden)
self.weights_ho = np.random.rand(self.hidden, self.outputs)
self.bias_h = np.random.rand(1, self.hidden)
self.bias_o = np.random.rand(1, self.outputs)
def feedforward(self, inputs):
self.hidden_layer = sigmoid(np.dot(inputs, self.weights_ih) + self.bias_h)
self.output_layer = sigmoid(np.dot(self.hidden_layer, self.weights_ho) + self.bias_o)
return self.output_layer
def backpropagate(self, inputs, targets, learning_rate):
# Calculate the error
error = targets - self.output_layer
# Calculate the gradient of the output layer
output_gradient = sigmoid_derivative(self.output_layer)
output_delta = error * output_gradient
# Calculate the gradient of the hidden layer
hidden_gradient = sigmoid_derivative(self.hidden_layer)
hidden_error = np.dot(output_delta, self.weights_ho.T)
hidden_delta = hidden_error * hidden_gradient
# Update the weights and biases
self.weights_ho += np.dot(self.hidden_layer.T, output_delta) * learning_rate
self.weights_ih += np.dot(inputs.T, hidden_delta) * learning_rate
self.bias_o += np.sum(output_delta, axis=0) * learning_rate
self.bias_h += np.sum(hidden_delta, axis=0) * learning_rate
SGD (Stochastic Gradient Descent)
- Stochastic Gradient Descent (SGD) is a variant of the gradient descent algorithm that is commonly used to train machine learning models.
- The algorithm works by updating the weights of the model using the gradient of the loss function with respect to a subset of the training data, rather than the entire dataset.
- SGD is particularly useful for large datasets or online learning scenarios, where it is computationally expensive to calculate the gradient of the entire dataset.
import numpy as np
# Define the loss function
def loss_function(y_true, y_pred):
return np.mean((y_true - y_pred)**2)
# Define the gradient of the loss function
def loss_gradient(y_true, y_pred):
return 2 * (y_pred - y_true)
# Define the Stochastic Gradient Descent algorithm
def sgd(X, y, model, learning_rate, epochs):
for _ in range(epochs):
for i in range(len(X)):
inputs = X[i]
target = y[i]
output = model.feedforward(inputs)
model.backpropagate(inputs, target, learning_rate)
Convnet
- Convolutional Neural Networks (ConvNets) are a class of deep learning models that are commonly used for image recognition and computer vision tasks.
- The architecture of a ConvNet consists of multiple layers, including convolutional layers, pooling layers, and fully connected layers.
- ConvNets are designed to automatically and adaptively learn spatial hierarchies of features from the input data, making them well-suited for tasks such as object detection and image classification.
import numpy as np
import tensorflow as tf
from tensorflow.keras import layers
# Define the ConvNet model
model = tf.keras.Sequential([
layers.Conv2D(32, (3, 3), activation='relu', input_shape=(28, 28, 1)),
layers.MaxPooling2D((2, 2)),
layers.Conv2D(64, (3, 3), activation='relu'),
layers.MaxPooling2D((2, 2)),
layers.Conv2D(64, (3, 3), activation='relu'),
layers.Flatten(),
layers.Dense(64, activation='relu'),
layers.Dense(10, activation='softmax')
])
# Compile the model
model.compile(optimizer='adam',
loss='sparse_categorical_crossentropy',
metrics=['accuracy'])
# Train the model
model.fit(X_train, y_train, epochs=10)
transformer
- The Transformer architecture is a deep learning model that is commonly used for natural language processing tasks such as machine translation and text generation.
- The architecture consists of an encoder-decoder structure with self-attention mechanisms that allow the model to focus on different parts of the input sequence.
- Transformers have achieved state-of-the-art performance on a wide range of NLP tasks and are known for their scalability and ability to capture long-range dependencies in the data.
import tensorflow as tf
from tensorflow.keras.layers import Input, Dense
from tensorflow.keras.models import Model
from tensorflow.keras.optimizers import Adam
from tensorflow.keras.losses import SparseCategoricalCrossentropy
# Define the Transformer model
def transformer_model(input_vocab_size, target_vocab_size, d_model, num_heads, dff, num_layers, dropout_rate):
inputs = Input(shape=(None,))
targets = Input(shape=(None,))
encoder = Encoder(input_vocab_size, d_model, num_heads, dff, num_layers, dropout_rate)
decoder = Decoder(target_vocab_size, d_model, num_heads, dff, num_layers, dropout_rate)
enc_output = encoder(inputs)
dec_output = decoder(targets, enc_output)
outputs = Dense(target_vocab_size, activation='softmax')(dec_output)
model = Model(inputs=[inputs, targets], outputs=outputs)
model.compile(optimizer=Adam(), loss=SparseCategoricalCrossentropy(), metrics=['accuracy'])
return model
Reinforce algorithm
- The REINFORCE algorithm is a policy gradient method used in reinforcement learning to train agents to maximize the expected cumulative reward.
- The algorithm works by estimating the gradient of the expected reward with respect to the policy parameters and updating the policy in the direction that increases the expected reward.
- REINFORCE is a simple and effective algorithm that is widely used in practice for training agents in a variety of environments.
import numpy as np
# Define the REINFORCE algorithm
def reinforce(env, policy, num_episodes, learning_rate, gamma):
for _ in range(num_episodes):
states, actions, rewards = [], [], []
state = env.reset()
done = False
while not done:
action = policy(state)
next_state, reward, done, _ = env.step(action)
states.append(state)
actions.append(action)
rewards.append(reward)
state = next_state
for t in range(len(states)):
G = sum([gamma**i * rewards[i] for i in range(t, len(rewards))])
policy.update(states[t], actions[t], learning_rate * G)
Diffusion model
- The Diffusion Model is a generative model used in machine learning to model the spread of information or influence in a network.
- The model works by simulating the diffusion process, where information is passed from one node to its neighbors in the network.
- Diffusion models are used in a wide range of applications, including social network analysis, recommendation systems, and epidemic modeling.
import networkx as nx
# Create a directed graph
G = nx.DiGraph()
# Add edges to the graph
G.add_edges_from([(1, 2), (2, 3), (3, 4), (4, 1)])
# Compute the Diffusion Model
diffusion = nx.diffusion_model(G)
print(diffusion)