Published
- 3 min read
AI Facts 1
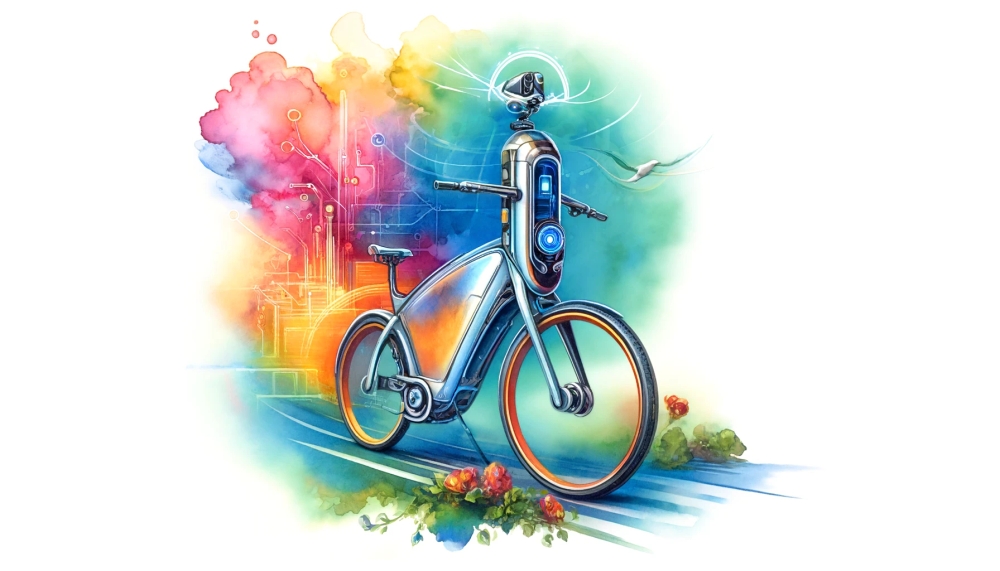
K-means Clustering
- K-means is a popular clustering algorithm that aims to partition
n
observations intok
clusters. - The algorithm works by iteratively assigning each data point to the nearest cluster and then recalculating the cluster centroids.
- The process continues until the centroids no longer change significantly or the maximum number of iterations is reached.
from sklearn.cluster import KMeans
import numpy as np
# Generate some data
X = np.array([[1, 2], [5, 8], [1.5, 1.8], [8, 8], [1, 0.6], [9, 11]])
# Create a KMeans instance
kmeans = KMeans(n_clusters=2)
# Fit the data
kmeans.fit(X)
# Get the cluster labels
labels = kmeans.labels_
# Get the cluster centroids
centroids = kmeans.cluster_centers_
print(labels)
print(centroids)
Spectral Clustering
- Spectral clustering is a graph-based clustering algorithm that uses the eigenvalues of a similarity matrix to reduce the dimensionality of the data.
- The algorithm works by constructing a similarity graph from the data points and then using the eigenvectors of the Laplacian matrix to partition the data into clusters.
- Spectral clustering is particularly useful for clustering data that is not linearly separable.
from sklearn.cluster import SpectralClustering
import numpy as np
# Generate some data
X = np.array([[1, 2], [5, 8], [1.5, 1.8], [8, 8], [1, 0.6], [9, 11]])
# Create a SpectralClustering instance
spectral = SpectralClustering(n_clusters=2)
# Fit the data
spectral.fit(X)
# Get the cluster labels
labels = spectral.labels_
print(labels)
FFT (Fast Fourier Transform)
- The Fast Fourier Transform (FFT) is an algorithm that computes the Discrete Fourier Transform (DFT) of a sequence or its inverse.
- The algorithm is widely used in signal processing, image processing, and data compression.
- The FFT algorithm has a complexity of
O(n log n)
, making it much faster than the naive DFT algorithm, which has a complexity ofO(n^2)
.
import numpy as np
# Generate some data
x = np.array([1.0, 2.0, 1.0, -1.0, 1.5])
# Compute the FFT
y = np.fft.fft(x)
print(y)
Random forest
- Random Forest is an ensemble learning method that constructs a multitude of decision trees at training time and outputs the class that is the mode of the classes (classification) or mean prediction (regression) of the individual trees.
- Random Forest is a popular algorithm due to its simplicity, scalability, and ability to handle high-dimensional data with high accuracy.
- Random Forest is robust to overfitting and can handle missing values and noisy data.
from sklearn.ensemble import RandomForestClassifier, GradientBoostingClassifier
from sklearn.datasets import make_classification
from sklearn.model_selection import train_test_split
from sklearn.metrics import accuracy_score
# Generate some data
X, y = make_classification(n_samples=1000, n_features=20, n_classes=2, random_state=42)
# Split the data into training and testing sets
X_train, X_test, y_train, y_test = train_test_split(X, y, test_size=0.2, random_state=42)
# Create a Random Forest classifier
rf = RandomForestClassifier(n_estimators=100, random_state=42)
# Fit the model
rf.fit(X_train, y_train)
# Make predictions
y_pred = rf.predict(X_test)
# Calculate accuracy
accuracy = accuracy_score(y_test, y_pred)
print(accuracy)
Gradient Boosting
- Gradient Boosting is an ensemble learning method that builds a series of weak learners (typically decision trees) and combines them to create a strong learner.
- The algorithm works by fitting a model to the data and then fitting subsequent models to the residuals of the previous models.
- Gradient Boosting is a powerful algorithm that can achieve high accuracy on a wide range of problems.
# Create a Gradient Boosting classifier
gb = GradientBoostingClassifier(n_estimators=100, random_state=42)
# Fit the model
gb.fit(X_train, y_train)
# Make predictions
y_pred = gb.predict(X_test)
# Calculate accuracy
accuracy = accuracy_score(y_test, y_pred)
print(accuracy)